Best Practices for Building an AI Data Strategy: A Guide for Mid-Sized Businesses
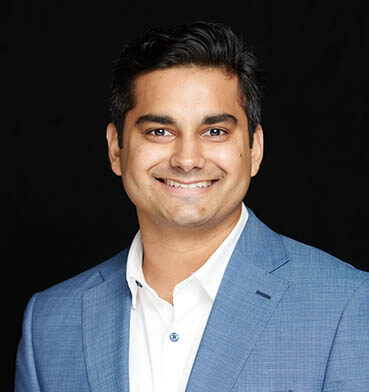
Poonit Mistry
Manager
Evolution Analytics, LLC.
Posted: November 27, 2024
As a mid-sized business leader, you’re likely hearing a lot about the potential of artificial intelligence (AI) to revolutionize operations, reduce costs, and boost revenue. But where do you start? Developing a solid AI data strategy is the foundation for reaping these benefits. At Evolution Analytics, we’ve worked with companies like yours to help define and implement AI strategies that deliver measurable impact. Here’s how we do it and how you can too.
Step 1: Define Clear Objectives and Understand Your Business Context
The first step in any successful AI journey is clarity. You need to start with a crystal-clear understanding of what you want to achieve and how it aligns with your business goals.
- Set Specific Objectives: AI isn’t a magic bullet. Its effectiveness hinges on being tied to well-defined business goals, like reducing customer churn, optimizing supply chains, or growing revenue through personalized marketing. With these goals in mind, define measurable KPIs to track your progress and the value AI delivers.
- Consider Integration and Change Management: AI doesn’t operate in a vacuum. To unlock its full potential, you’ll need to assess how predictions will integrate into existing business processes and systems. This means engaging stakeholders early and planning for workflow adjustments. AI’s success often depends more on how it’s used than on how it’s built.
Step 2: Build Practical Development and Deployment Strategies
An AI strategy needs to balance ambition with pragmatism. Start small and iterate.
- Start with a Proof of Concept (POC): The quickest way to prove value is by selecting a manageable use case and developing a small-scale POC. This approach lets you test assumptions, refine your models, and build internal confidence.
- Leverage Existing Infrastructure: You likely already have valuable resources at your disposal—whether it’s data warehouses like Snowflake, cloud platforms like AWS or Azure, or tools like Jupyter notebooks. These technologies can reduce the cost and complexity of scaling AI initiatives.
Step 3: Foster Collaboration and Plan for Continuous Improvement
AI is a team sport. Success depends on fostering collaboration across various roles and continuously refining your solutions.
- Promote Cross-Functional Collaboration: Building AI solutions requires a diverse set of skills. Data scientists, engineers, MLOps professionals, and business stakeholders all bring unique expertise to the table. Creating a culture of collaboration ensures that your AI solutions are both technically sound and business-relevant.
- Plan for Ongoing Improvements: AI doesn’t end at deployment. Models need to evolve with your business and external conditions. Incorporating CI/CD pipelines can streamline updates, while regular retraining, hyperparameter tuning, and A/B testing ensure your models stay accurate and effective.
Step 4: Build Strong Data Capabilities and Governance
AI is only as good as the data powering it. Robust data management practices and governance frameworks are essential.
- Ensure Data Quality and Accessibility: AI models thrive on accurate, consistent, and clean data. Breaking down silos and integrating data from various sources provides a comprehensive view of your business, while data democratization ensures timely access for decision-makers.
- Prioritize Governance, Security, and Privacy: With great power comes great responsibility. Establish clear policies for data access, usage, and protection, and ensure compliance with relevant regulations. For example, maintaining data lineage provides transparency, while techniques like Retrieval-Augmented Generation (RAG) improve accuracy and reduce risks like AI hallucinations.
- Mitigate Key AI Risks: Alongside governance, it’s crucial to address potential pitfalls, such as:
- Bias in AI Models: AI can inadvertently reinforce biases in your data, leading to unfair or inaccurate outcomes. Regularly audit your models and datasets to identify and mitigate potential biases.
- Ethical Concerns: AI applications can pose ethical dilemmas, particularly in areas like surveillance or automated decision-making. Engage stakeholders to ensure ethical considerations are embedded into your AI strategy.
- Deployment Failures: Misaligned expectations, inadequate testing, or insufficient infrastructure can lead to failed deployments. A phased, iterative approach with rigorous testing minimizes the risk of costly errors.
What Makes Evolution Analytics Different?
When you partner with us, you’re not just getting tools—you’re gaining a team of experts who guide you through every stage of your AI journey.
- Tailored Strategies: We take the time to understand your business, crafting AI strategies that align with your goals and challenges.
- Proven Technology Expertise: From Snowflake to advanced AI/ML tools, we help you maximize your existing infrastructure and invest in scalable, future-ready solutions.
- Continuous Support: Beyond initial deployment, we help you refine your models, manage your data governance, and scale your AI initiatives to meet growing demands.
Let’s Get Started
Developing an AI data strategy doesn’t have to be overwhelming. By following these best practices and partnering with a trusted expert, you can unlock the power of AI to drive meaningful business outcomes. At Evolution Analytics, we’re here to guide you every step of the way. Ready to transform your business with AI? Let’s talk.